Why Data Analysis Matters in the Insurance Industry
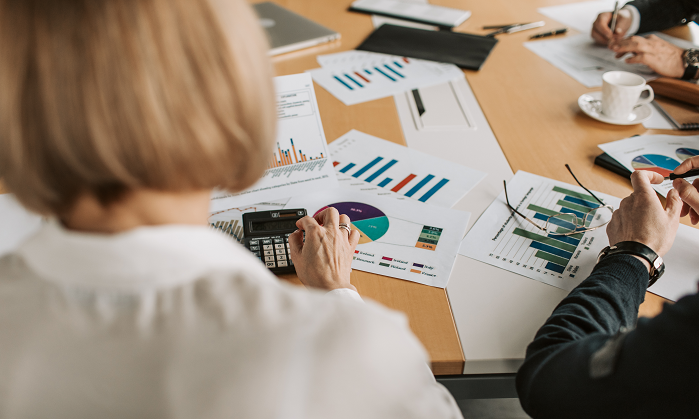
Table of content
Overview in Data Analytics in Insurance
What are the main ethical concerns regarding the use of AI in insurance analytics?
Different ways Digital and Analytics in Insurance
Use Cases of Insurance Data Analytics in Different Industry
Conclusion
Overview in Data Analytics in Insurance
The insurance industry is evolving rapidly, with a growing array of options available to clients. This abundance can be overwhelming as people grapple with questions about a company’s safety, value, and market reputation. Insurers face their own challenges, such as understanding customer behavior, managing fraud, and assessing policy risks. While many companies have made strides in selling directly online and competing on price comparison sites, achieving this isn’t always easy.
Data prefiltration and advanced mathematical models have long been essential in insurance, helping to analyze customer behavior and risk costs. Traditionally, companies relied on historical data through descriptive analytics. However, there’s a growing demand for more forward-looking insights—predictive analytics to forecast future trends and prescriptive analytics to determine how actions can influence outcomes.
Big data has transformed the insurance sector into an ideal playground for analytics, revealing patterns and offering insights to navigate the intricate relationships between agents and clients.
What are the main ethical concerns regarding the use of AI in insurance analytics?
Privacy and Data Protection:
Collect, store, and use customer data responsibly, following regulations like GDPR.
Implement strong security measures to safeguard against data breaches and unauthorized access.
Secure explicit consent from customers for how their personal data will be used.
Algorithmic Fairness and Bias Mitigation:
Identify and address biases in the data and algorithms used for assessing risk and setting prices.
Ensure that insurance decisions do not unfairly discriminate based on race, gender, or socioeconomic status.
Conduct regular audits to ensure that algorithms remain fair and transparent.
Transparency and Explainability:
Offer clear explanations to customers about how AI-based decisions are made and what factors are involved.
Allow customers to understand and challenge automated decisions that affect them.
Make sure AI decision-making processes are transparent and can be reviewed.
Ethical Data Practices:
Follow principles of data minimization and use data only for the intended purpose.
Avoid repurposing customer data for uses beyond its original intent.
Create and enforce policies and governance structures to ensure the ethical use of data and AI.
Accountability and Oversight:
Clearly define roles and responsibilities for developing, deploying, and monitoring AI systems.
Set up external oversight and auditing mechanisms to review AI practices in insurance.
Develop procedures to address and correct any unintended consequences or issues caused by AI.
Different ways Digital and Analytics in Insurance
Here are the key ways digital technologies and analytics are transforming the insurance industry:
Improved Customer Experience
Digital self-service tools, such as online portals and mobile apps, offer customers round-the-clock access to information and services, making their experience more convenient and satisfying. Personalized recommendations tailored to individual needs and preferences further enhance their overall experience.
Optimized Underwriting and Risk Assessment:
Advanced analytics provide more precise risk evaluation by examining factors like income, age, medical history, vehicle type, and property details. Natural language processing helps verify customer information from various digital sources, offering a comprehensive view of risk. Real-time risk scoring and premium calculations become feasible with these analytics.
Accelerated Claims Management:
Analytics streamline the claims process by automating tasks like prioritization and payouts, using historical data and loss types. Advanced APIs facilitate situation-specific responses and effective information extraction.
Enhanced Fraud Detection:
Machine learning algorithms in predictive analytics identify potential fraudulent claims by cross-referencing customer history, helping to detect fraud more effectively.
Personalized Product Development:
Analytics deliver insights into what customers want, allowing insurers to focus on developing products that meet specific needs. This targeted approach helps in refining offerings based on user behavior and preferences.
Streamlined Operations:
Automating manual tasks such as underwriting and claims processing cuts costs and boosts employee productivity. Digital technologies also help shorten the cycle time for settling claims.
Data-Driven Insights:
An enterprise data platform enables large-scale data analysis to uncover insights for innovative product development. Real-time, accurate data shared across the organization supports informed decision-making and enhances performance.
Use Cases of Insurance Data Analytics in Different Industry
- Insurance Pricing:
Insurance companies often face worsening underwriting results, especially if they rely on outdated methods that lead to mispricing. With the ability to compare prices easily in today’s market, customers often choose the lowest option, which may not always align with the actual risk involved. This can end up costing companies significantly.
User
Automated tools for building and comparing pricing models help users determine if a risk is priced appropriately. Dashboards highlight the top three companies with the most customers and those offering the lowest prices, making it easier for customers to find the best insurance deals. For example, the bar chart reveals that SBI Life Insurance is popular among the 10 to 20 age group. - Claim Payment Automation Modeling:
Manual inspections for insurance claims often lead to delays in payouts, which can spike claim amounts, harm customer satisfaction, and reduce retention rates.
User
Dashboards display the top five policies with the highest customer investments and the most lucrative age groups, helping insurance companies understand demand. Faster claim processing boosts customer satisfaction and helps in accurately reserving amounts for incurred but not reported (IBNR) losses. This leads to more reliable pricing models based on predicted losses. - Claim Development Modeling:
Claim amounts can vary significantly from the initial filing to the final payment. Accurate predictions of these final amounts are crucial for financial statements and reserve management.
User
Building precise, automated predictive models helps in forecasting the ultimate cost of claims. Dashboards show which policies attract the most customers across different age groups and track claim trends over the year, aiding in future predictions and tailored recommendations. - Life Insurance for Impaired Life Customers:
Underwriting customers with serious illnesses usually requires extensive and costly medical assessments.
User
By analyzing medical histories and conditions, life reinsurers can more accurately assess the risk of underwriting customers with serious diseases. This approach helps insurers directly underwrite healthier customers, reducing medical costs and expanding their client base. For instance, data shows that clients with advanced stages of blood cancer have higher mortality risks, but trends indicate that the death rate decreases over time, making it safer to offer coverage to these patients. - Fraudulent Claim Modeling:
Investigating every claim for fraud is expensive and often inefficient, and wrongly accusing innocent customers can drive them away.
User
Predictive modeling helps identify and prioritize potential fraudulent claims. Dashboards reveal which age groups and occupations are most associated with fraud, allowing insurers to focus resources effectively and improve customer satisfaction by avoiding wrongful accusations.
Our solutions are designed to adapt to the dynamic needs of various industries. Click here to learn more about our Big Data Testing Services and Solutions.
Conclusion
In conclusion, the insurance industry is evolving with advanced data analytics and digital technologies that improve customer experiences and streamline operations. From enhancing underwriting and risk assessment to accelerating claims management and detecting fraud, these innovations offer significant benefits. However, they also bring ethical challenges like privacy and fairness that must be addressed. Embracing these technologies responsibly enables insurers to provide more accurate pricing, faster claims processing, and better service. Discover how our Big Data Testing Services can support your success in this dynamic industry.
Get your data results fast and accelerate your business performance with the insights you need today.