Power of Predictive Maintenance with Machine Learning
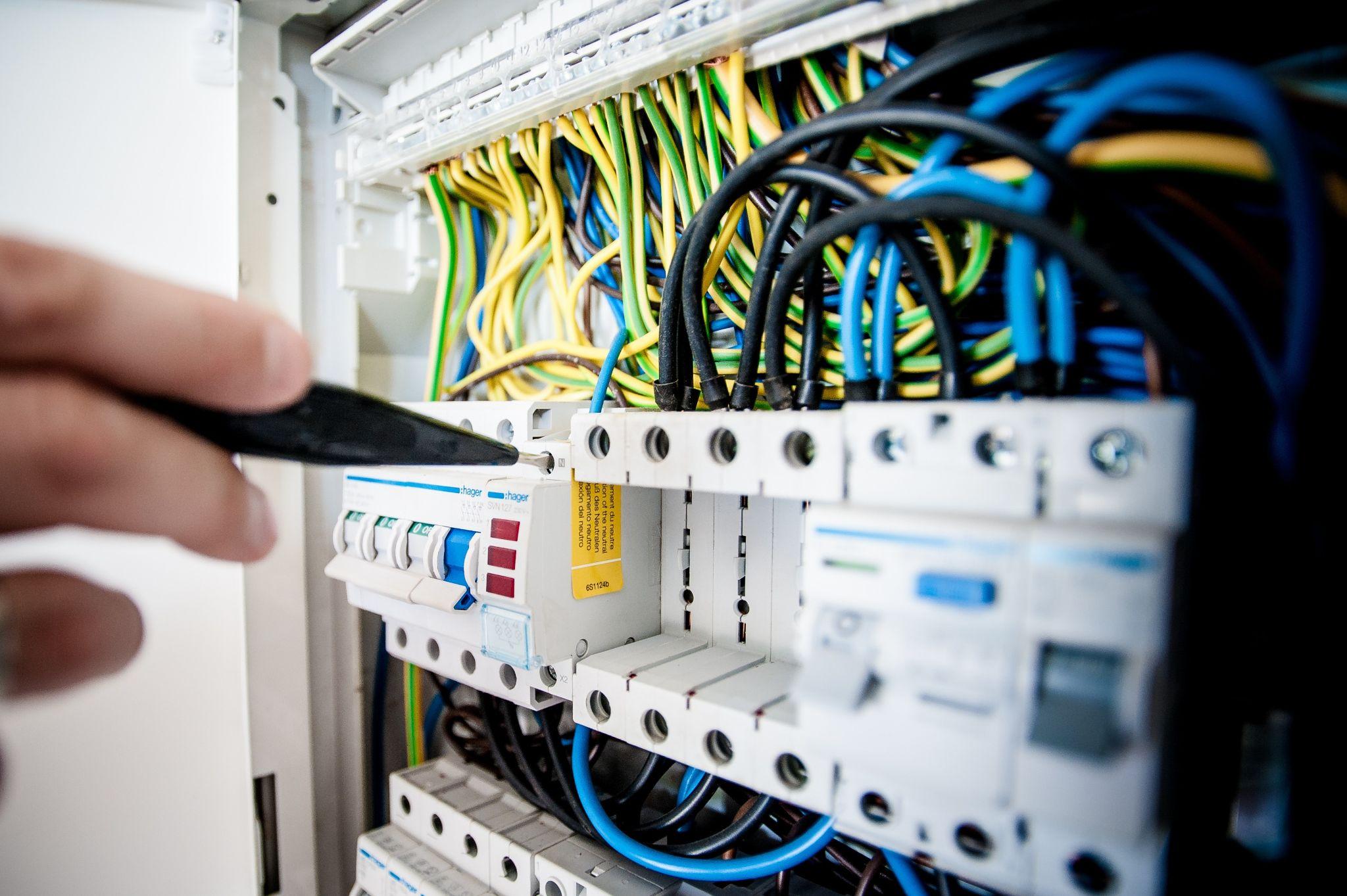
Table of content
What is Predictive Maintenance?
Predictive Maintenance: How it Works
Difference Between Preventive and Predictive Maintenance
What is Preventive Maintenance?
What is Predictive Maintenance?
What is Predictive Maintenance?
Predictive maintenance is an advanced approach that builds upon condition-based monitoring to enhance both the performance and lifespan of equipment. It does this by continuously evaluating the health of machinery in real-time.
By gathering data from sensors and using advanced analytical techniques like machine learning (ML), predictive maintenance can not only detect and address problems as they arise but also forecast future issues. This reduces risks and ensures equipment stays in optimal condition. The key to its success lies in delivering the right information to the right people at the right time.
Predictive Maintenance : How it works
Predictive maintenance (PdM) leverages intelligent algorithms to accurately predict the remaining lifespan of machinery and its components. By combining various data sources with real-time hardware analysis, predictive maintenance enables businesses to move away from scheduled maintenance and adopt usage-based strategies. With predictive maintenance using machine learning, the service schedule adapts to the actual condition and usage of the equipment.
For example, in manufacturing, PdM can predict equipment malfunctions by analyzing vibration and temperature sensor data, allowing proactive interventions that prevent costly downtimes. Similarly, in aviation, PdM forecasts engine issues based on historical performance data, enabling airlines to carry out maintenance during non-operational hours, thus minimizing disruptions to flight schedules. These examples demonstrate how predictive maintenance and predictive maintenance using machine learning are transforming maintenance practices in various industries by optimizing asset performance and cutting operational costs.
Difference Between preventive and predictive maintenance
Both preventive maintenance and predictive maintenance aim to enhance asset reliability and minimize unexpected breakdowns. While both are forms of scheduled maintenance, they differ in how they are applied.
With preventive maintenance, tasks are carried out at regular intervals, regardless of the equipment’s condition. In contrast, predictive maintenance is based on real-time asset conditions, ensuring repairs or adjustments are made only when necessary. While preventive maintenance is less costly to implement, predictive maintenance has the advantage of reducing both labor and material costs by addressing issues as they arise.
However, predictive maintenance requires a significant initial investment in terms of money, training, and resources. Despite these upfront costs, many companies find that once a predictive maintenance program is in place, it pays off in the long run by minimizing unexpected failures and reducing operational costs.
What is Preventive Maintenance?
Think of preventive maintenance like a routine health check-up. Technicians perform these tasks even when equipment is functioning properly to avoid future problems. Similar to how regular doctor visits can help prevent illness, preventive maintenance helps avoid equipment breakdowns and extends the life of assets. Keeping production lines running smoothly leads to greater profitability.
The challenge with preventive maintenance lies in balancing the costs with the benefits. Skilled maintenance managers need to make informed decisions about which equipment requires attention and how often.
What is Predictive Maintenance?
While predictive maintenance shares similarities with preventive maintenance, it focuses on specific conditions. In a health analogy, it’s like screenings for individuals who are at higher risk for certain illnesses. If equipment starts behaving unusually, technicians initiate predictive maintenance protocols to schedule repairs or address potential failures before they happen.
To make this process more efficient, investing in a computerized maintenance management system is highly recommended. Such a system provides the data needed to make informed decisions, reducing unnecessary tasks and maintenance costs, while ensuring equipment runs smoothly.
Just as a healthy lifestyle, regular exercise, and medical exams contribute to overall well-being, both preventive and predictive maintenance play crucial roles in maintaining a well-functioning facility.
Key Differences between Preventive and Predictive Maintenance
The key difference between the two is timing: preventive maintenance follows a fixed schedule, while predictive maintenance is performed only when necessary, based on asset conditions. Though predictive maintenance can save on labor and materials, it requires a larger upfront investment in technology and resources. However, for organizations already benefiting from a preventive maintenance program, the transition to predictive maintenance can be a highly effective next step.
How Is Predictive Maintenance Using Machine Learning?
In simple terms, predictive maintenance (PdM) uses intelligent algorithms, artificial intelligence, and data analytics to predict the remaining lifespan of machines and their components. By combining multiple data sources with real-time or near-real-time analysis, PdM enables businesses to monitor equipment conditions continuously. With the help of built-in intelligent sensors like vibration, temperature, and electric current sensors, and using both local device processing and cloud computing, PdM identifies potential problems before they lead to failure. The local or remote software constantly analyzes the readings to assess machine conditions.
With advanced modeling technologies and predictive maintenance using machine learning, the system elevates asset monitoring by examining hundreds of process parameters over time and comparing them to historical data. This process provides operators with early, more accurate information, allowing them more time to resolve issues and avoid costly failures.
Benefits of Machine Learning in Maintenance Optimization
The rising popularity of predictive maintenance using machine learning is driven by its ability to generate significant cost savings. By performing manual inspections only when necessary, companies can reduce expenses and minimize the downtime caused by unnecessary equipment shutdowns. Machine learning offers sophisticated tools for predicting equipment failures and optimizing maintenance schedules. Supervised learning algorithms, such as regression and classification, analyze historical data to identify patterns indicating potential failures, which leads to proactive interventions. In contrast, unsupervised learning algorithms, like clustering and anomaly detection, scan large datasets to uncover irregularities or outliers that signal potential issues.
Moreover, reinforcement learning allows for dynamic maintenance scheduling, where strategies are adjusted based on real-time feedback, optimizing the use of resources. By using this combination of machine learning techniques, businesses can precisely predict equipment failures, streamline maintenance processes, reduce downtime, and maximize efficiency.
Implementing Predictive Maintenance Using Machine Learning
The primary goals when implementing predictive maintenance using machine learning are to improve operational efficiency, reduce downtime, and achieve financial savings. For a successful implementation, it’s essential to develop a strategic approach, including the following steps:
Data Acquisition and Preprocessing
Data acquisition and preprocessing are fundamental to ensuring the success of predictive maintenance using machine learning. Quality, relevant, and consistent data are critical to training predictive models effectively. By optimizing data quality, companies can improve equipment reliability, minimize downtime, and reduce maintenance costs. Here’s how to approach it:
- A systematic data collection method should be established, utilizing Internet-of-Things (IoT) sensors, SCADA systems, and other monitoring devices to gather real-time data from production sources.
- The data should include sensor readings, equipment logs, maintenance records, and environmental factors relevant to predictive maintenance.
- Preprocessing the data involves filtering out outliers and normalizing data ranges to standardize the dataset for analysis. This step ensures that the data is consistent and ready for model training.
- Maintaining data quality and integrity throughout the process ensures the predictive models remain reliable, forming the foundation for effective maintenance strategies.
Model Training and Evaluation
For maintenance managers overseeing the implementation of predictive maintenance using machine learning, proper model training and evaluation are crucial. Here are some key points:
- Begin by selecting suitable algorithms, whether they involve regression, classification, clustering, or reinforcement learning, and train models using preprocessed data.
- Utilize model evaluation techniques such as cross-validation and metrics like accuracy, precision, recall, and F1-score to assess the models’ performance.
- Ensure that evaluation metrics align with your KPIs, such as minimizing downtime, extending equipment lifespan, and reducing maintenance costs. This alignment helps gauge the overall success of predictive maintenance initiatives.
Implementation Challenges and Solutions
Before integrating PdM into regular operations, companies must address common challenges such as imbalanced datasets, data drift, model interpretability, and scalability issues. Techniques like oversampling or undersampling can mitigate imbalanced datasets, while continuous monitoring helps manage data drift, ensuring model accuracy over time.
Constantly monitoring and updating predictive maintenance models using machine learning is vital to ensuring continued success. Real-time monitoring of equipment conditions enables timely interventions, and updating ML models with new data ensures continuous optimization, helping companies maintain operational efficiency and prevent unexpected failures.
Get your data results fast and accelerate your business performance with the insights you need today.