Mystery of AI Decisions: Exploring World of Explainable AI
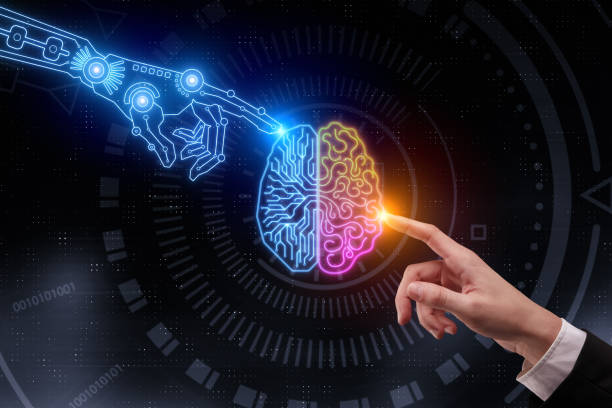
Artificial intelligence (AI) has become an integral part of our daily lives, from personalised shopping recommendations to medical diagnoses. However, as AI models become more complex, it becomes increasingly difficult to understand how these models arrive at their decisions. This can be a significant obstacle for businesses and organisations that need to explain AI decisions to their customers or regulators. In this article, we explore the concept of explainable AI (XAI) and its importance in various industries. We also discuss some of the current and potential XAI techniques, such as transparency, interpretability, and causality, and their benefits and limitations.
The Need for Explainable AI
AI decision-making is often perceived as a “black box,” meaning it is unclear how the AI models arrive at their decisions. This opacity is a significant obstacle to trust and accountability, which is necessary for the adoption of AI in various industries. The inability to understand AI decisions can lead to incorrect conclusions, erroneous actions, and unintended consequences. In many cases, it may be necessary to explain AI decisions to customers or regulators. For example, in the healthcare industry, it may be necessary to explain how an AI model arrived at a medical diagnosis to the patient or a regulatory authority. Without this explanation, it is difficult to build trust in AI systems and ensure their adoption.
Explainable AI can help overcome these obstacles. XAI is the practice of designing AI models that can provide explanations for their decisions. This makes AI decision-making more transparent and understandable, enabling businesses and organisations to explain their decisions to customers or regulators. It also helps to identify and correct errors in AI decision-making, improving the overall accuracy and effectiveness of AI models.
Techniques in Explainable AI
There are several techniques used in explainable AI, each with its benefits and limitations. These techniques can be broadly categorised into three categories: transparency, interpretability, and causality.
A. Transparency Techniques
Transparency techniques involve exposing the internal workings of an AI model to improve its understandability. These techniques can include:
Model Inspection: This involves analysing the AI model’s internal structure and behaviour to gain insight into how it makes decisions. This can be done by visualising the model’s architecture, weights, and activations.
Data Provenance: This involves tracking the origin and transformation of data used by the AI model. It helps to ensure that the data is accurate and unbiased, and it can help to identify errors or inconsistencies in the data.
B. Interpretability Techniques
Interpretability techniques involve generating explanations for AI model decisions. These explanations can help users understand how the AI model arrived at a particular decision. These techniques can include:
Feature Importance: This involves identifying the input features that had the most significant impact on the AI model’s decision. This can help users understand which features were most important in making the decision.
Attribution: This involves identifying the parts of the input data that contributed the most to the AI model’s decision. It can help users understand which parts of the input data were most influential in the decision-making process.
C. Causality Techniques
Causality techniques involve generating counterfactual explanations that help users understand how changes in input data would affect the AI model’s decision. These techniques can include:
Counterfactuals: This involves generating hypothetical scenarios in which input data is changed to see how it would affect the AI model’s decision. It can help users understand how sensitive the model is to changes in input data.
Inference: This involves inferring causal relationships between input data and AI model decisions. It can help users understand how different input features relate to the AI model’s decision.
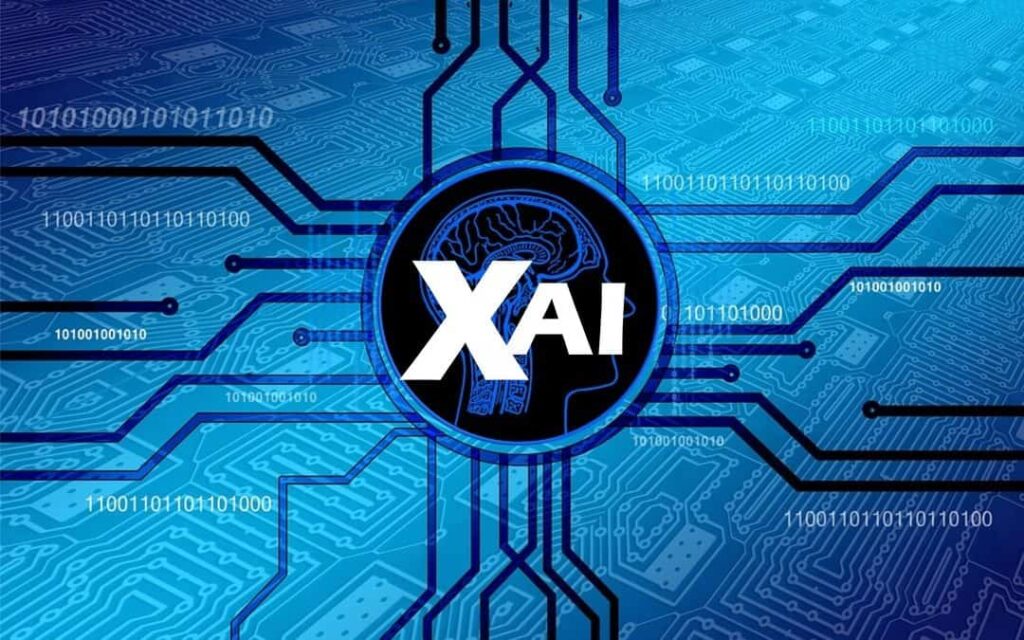
D. Comparison and Trade-offs among the Techniques
Each of these techniques has its benefits and limitations, and the choice of technique depends on the specific use case and the goals of the AI model. For example, transparency techniques can be useful for identifying biases in AI models, while interpretability techniques can be useful for understanding how the model arrived at a particular decision. Causality techniques can be useful for exploring hypothetical scenarios and understanding the relationship between input data and AI model decisions. It’s important to note that there are trade-offs between the different techniques. Some techniques may be more computationally expensive, while others may provide less insight into the AI model’s decision-making process.
Applications of Explainable AI
Explainable AI has many applications in various industries. Here are some examples:
A. Healthcare and Medical Diagnosis: XAI can help healthcare professionals understand how AI models arrive at medical diagnoses. This can lead to better patient outcomes, as healthcare professionals can make more informed decisions.
B. Finance and Risk Assessment: XAI can help financial institutions explain their risk assessment decisions to regulators and customers. This can lead to more trust and transparency in the financial industry.
C. Autonomous Driving and Robotics: XAI can help designers of autonomous vehicles and robots understand how their AI models make decisions. This can improve the safety and reliability of these systems.
D. Cybersecurity and Fraud Detection: XAI can help cybersecurity professionals understand how AI models detect and respond to cyber threats. This can lead to more effective cybersecurity measures and better fraud detection.
Current Challenges and Future Directions
While XAI has many benefits, there are also challenges associated with its implementation. One challenge is that some XAI techniques can be computationally expensive, which can be a barrier to adoption. Another challenge is that XAI can sometimes be difficult to interpret, which can lead to incorrect conclusions. Additionally, there is a risk that XAI could perpetuate existing biases in AI decision-making.
To address these challenges, interdisciplinary research and collaboration are necessary. Researchers in computer science, psychology, and ethics must work together to develop XAI techniques that are effective, efficient, and ethical. Additionally, there must be a focus on developing XAI techniques that are accessible to non-experts, so that businesses and organisations can effectively utilise XAI.
Conclusion
Explainable AI is an essential component of responsible AI development and deployment. By providing transparency, interpretability, and causality, XAI can help businesses and organisations build trust in AI models and improve their effectiveness. However, there are challenges associated with XAI implementation, and interdisciplinary collaboration is necessary to overcome these challenges. With continued research and development, XAI has the potential to transform the way we use AI and improve our understanding of complex decision-making processes.
Get your data results fast and accelerate your business performance with the insights you need today.