What is Data Wrangling ? Strategies for Cleaning and Preparing Data for Analysis
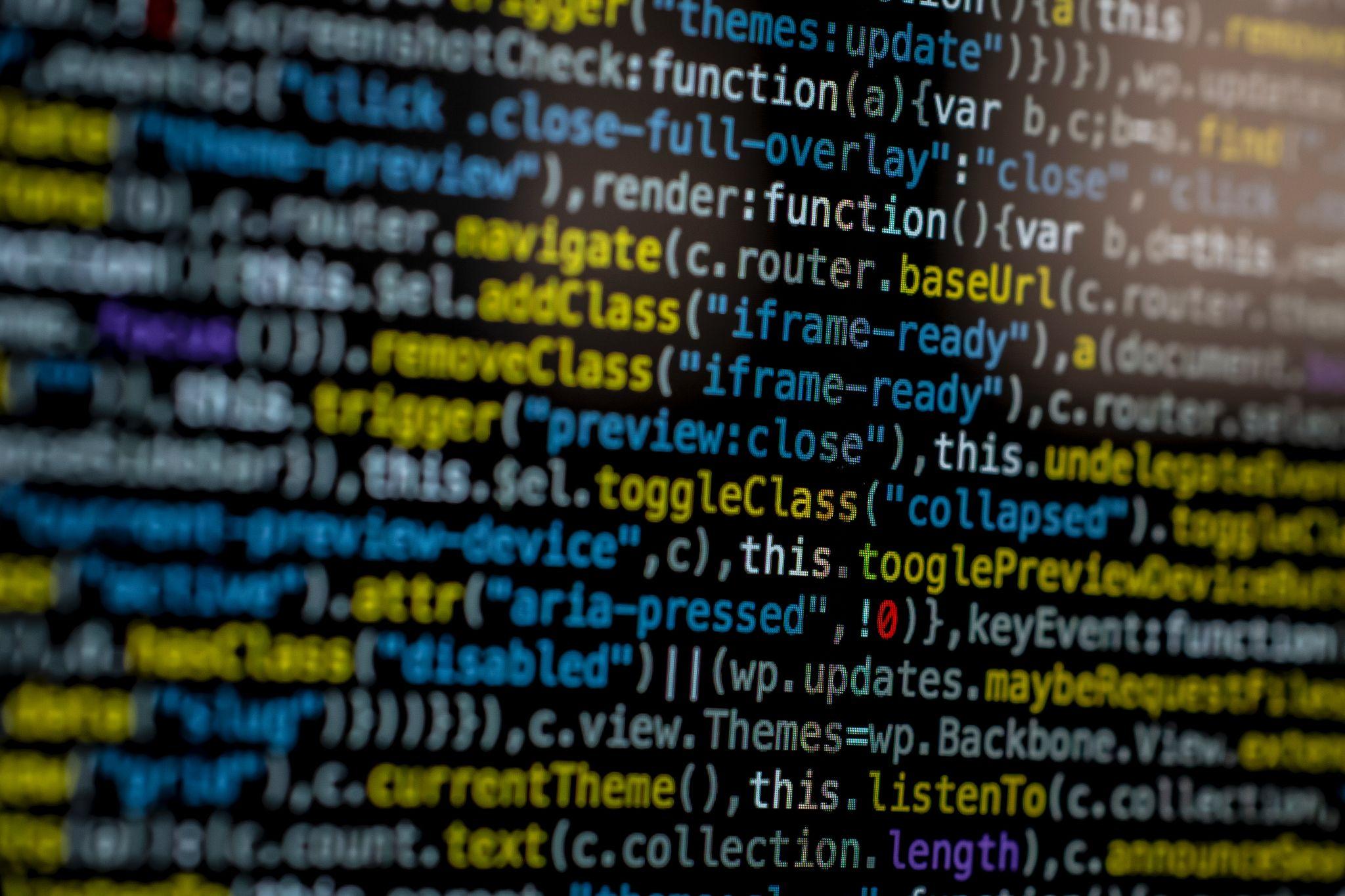
Table of content
What is Data Wrangling?
The Importance of Data Wrangling in 2024
How Data Wrangling Works?
Benefits of Data Wrangling
The Future of Data Wrangling
Conclusion
In today’s world, we’re constantly surrounded by a flood of information. Each piece of data is like a drop in a vast ocean, holding the potential to guide better decisions and drive a company’s success. The magic lies in how we unlock this potential, and that’s where data wrangling comes in. This essential process transforms raw data into a format that’s easier to analyze and derive insights from. But let’s be honest—data wrangling can feel like a daunting challenge. That’s why having a clear understanding and a well-structured approach is so important to make the process more manageable and effective.
What is Data Wrangling ?
Data wrangling, also known as data munging, is a vital process in the data analytics workflow that involves cleaning, structuring, and enriching raw data to make it more suitable for analysis. In the context of data wrangling in data science, this means removing or correcting inaccuracies, inconsistencies, and duplicates to ensure the data’s integrity. It also entails organizing the data into a structured, often tabular format, which makes it easier to work with in various analytical applications.
Enriching the data is another critical step in data wrangling, where additional information is incorporated to enhance its usefulness for analysis. This enriched data is then validated to confirm its accuracy and quality. Ultimately, data wrangling transforms raw data into a more accessible and meaningful form, enabling analysts and data scientists to derive valuable insights more efficiently and accurately.
The Importance of Data Wrangling in 2024
In 2024, the significance of data wrangling has become more critical than ever for several key reasons:
Volume and Variety of Data:
The rapid expansion of data from the internet, social media, IoT devices, and numerous other sources has led to a massive increase in the volume and variety of data that organizations must manage and analyze. Data wrangling plays a crucial role in efficiently processing this vast and diverse data landscape.
Advanced Analytics and AI:
The ongoing advancements in analytics and artificial intelligence (AI) rely heavily on high-quality data. Data wrangling ensures that the data entering these sophisticated models is clean, accurate, and well-structured, which is essential for the success of AI and machine learning initiatives.
Faster Decision-Making:
In today’s fast-paced business environment, the ability to make swift and informed decisions is vital for maintaining a competitive edge. Data wrangling speeds up data preparation, enabling organizations to quickly analyze data and gain valuable insights.
Compliance and Data Governance:
With increasing data privacy and usage regulations like GDPR and CCPA, organizations must ensure that their data is correctly handled and processed. Data wrangling plays a critical role in ensuring compliance by cleaning and structuring data in accordance with these regulatory standards.
Enhanced Data Quality and Accuracy:
The reliability of data analytics heavily depends on the quality and accuracy of the underlying data. Data wrangling significantly improves data quality and accuracy, thereby enhancing the trustworthiness of the insights derived from it.
How Data Wrangling Works?
Data wrangling is a multi-step process that transforms raw data into a format ready for analysis, making it essential for deriving valuable insights that drive decision-making and strategic planning. Here’s an in-depth look at the stages of data wrangling:
- Data Collection:
The initial stage involves gathering raw data from various sources, including databases, files, external APIs, web scraping, and other data streams. This data can be structured (e.g., SQL databases), semi-structured (e.g., JSON, XML files), or unstructured (e.g., text documents, images). - Data Cleaning:
After collection, data cleaning begins, a crucial step to eliminate errors, inconsistencies, and duplicates that could distort analysis. Cleaning involves removing irrelevant data, correcting errors, handling missing values, and resolving inconsistencies such as date or currency format variations. - Data Structuring:
Following cleaning, data is structured or restructured to facilitate analysis. This often involves converting unstructured or semi-structured data into a structured format, like a table or CSV file. Tasks may include parsing data into fields, normalizing formats, and transforming data for analysis readiness. - Data Enriching:
Data enrichment enhances the dataset by adding context or additional information, making it more valuable for analysis. This may involve merging data from multiple sources or creating new variables that offer deeper insights. - Data Validating:
Validation checks ensure data accuracy and quality post-cleaning, structuring, and enrichment. This step includes data integrity checks and quality assurance testing to confirm the data meets established standards. - Data Storing:
The final, processed data is stored in a data repository like a database or data warehouse, ensuring it is secure and easily accessible for future analysis and reporting. - Documentation:
Throughout the data wrangling process, documentation is vital. It records all transformations and decisions, supporting reproducibility, auditing, and a clear understanding of the data analysis process.
Benefits Of Data Wrangling ?
Data wrangling is a critical component of the data analytics process, offering a wide range of benefits that significantly elevate the value of data for businesses and organizations. By transforming raw data into a structured and clean format, data wrangling enhances the accuracy, efficiency, and insightfulness of subsequent analysis. Here’s how data wrangling can benefit your organization:
1. Improved Data Quality and Consistency:
Data wrangling addresses issues such as missing values, outliers, and inconsistencies, leading to a substantial improvement in overall data quality. By standardizing formats, units, and structures, it ensures consistency across datasets, making data integration more seamless and reliable.
2. Enhanced Analytical Efficiency and Support for EDA:
Systematic cleaning and transformation of data reduce the time and effort required for downstream analysis. Well-wrangled datasets enable more efficient Exploratory Data Analysis (EDA), allowing analysts to focus on identifying patterns and exploring relationships within the data.
3. Facilitated Data Integration and Adaptability:
Data wrangling enables the integration of information from diverse sources, creating a comprehensive dataset that provides a holistic view of the data. The process is adaptable to changes in data sources or formats, ensuring your data strategy remains robust as business needs evolve.
4. Preparation for Machine Learning and Enhanced Reproducibility:
For machine learning models to perform effectively, they require clean, structured input data. Data wrangling ensures that your models receive high-quality data, leading to more accurate predictions. Additionally, clear documentation of the wrangling process enhances reproducibility, ensuring transparency and facilitating the replication of analyses.
5. Supports Informed Decision-Making and Reduces Errors:
Reliable, well-organized data is the foundation of informed decision-making. By systematically addressing data quality issues, data wrangling reduces errors in analysis and reporting, resulting in more accurate and trustworthy insights.
6. Scalability for Growing Data Demands:
As your organization grows and the volume of data increases, data wrangling processes can scale accordingly. Effective data wrangling practices ensure that you can manage larger datasets without compromising data quality or the integrity of insights.
The Future of Data Wrangling
The future of data wrangling is poised to be both promising and transformative, as it evolves alongside technological advancements and the increasing significance of data in decision-making processes. Understanding what is data wrangling and its role in data science is essential as several key trends and developments are expected to shape its future:
Automation and Machine Learning:
With AI and machine learning technologies becoming more prevalent in data wrangling in data science, the automation of data preparation processes will accelerate. This evolution aims to minimize the time and effort required for data cleaning and transformation, enabling data scientists and analysts to focus on strategic tasks.
Increased Integration:
As businesses leverage a wider range of data sources, the integration capabilities of data wrangling tools will expand. This will lead to better and more seamless integration with databases, cloud storage, and various data formats, creating a more efficient data pipeline from ingestion to insights.
User-Friendly Interfaces:
To make data wrangling more accessible, particularly for those without extensive programming skills, tools will feature more intuitive graphical user interfaces. These advancements will democratize data analysis, allowing more individuals within organizations to engage in data-driven decision-making.
Real-time Data Processing:
As the demand for real-time analytics grows, data wrangling tools will increasingly support real-time data processing. This capability will be vital for applications that require immediate insights, such as fraud detection, live customer feedback analysis, and operational performance monitoring.
Data Quality and Governance:
As organizations become more data-driven, the emphasis on data quality and governance will grow. Data wrangling tools will integrate more features to automatically detect data quality issues and enforce data governance policies, ensuring that the data used for analysis is accurate, consistent, and compliant with regulations.
Collaboration Features:
The future of data wrangling will include enhanced collaboration features, enabling teams to work together more effectively on data preparation tasks. These features will include shared workspaces, version control, and the ability to track and review changes made by different team members.
Advanced Analytics Integration:
Data wrangling tools will increasingly incorporate or integrate with advanced analytics capabilities, such as predictive modeling and machine learning algorithms. This integration will streamline the workflow from raw data to actionable insights, allowing users to move seamlessly from data preparation to analysis.Focus on Privacy and Security:
As global data privacy regulations tighten, data wrangling tools will need to incorporate robust security measures and compliance mechanisms. This will involve ensuring that data is anonymized or encrypted as required and that the tools adhere to regulations like GDPR and CCPA.
Conclusion :
Data wrangling is a critical process that transforms raw data into a clean, structured, and enriched format, making it essential for effective data analysis in today’s information-driven world. As the volume and variety of data continue to grow, and as advanced analytics and AI become more integral to business strategies, the importance of data wrangling will only increase. By improving data quality, facilitating integration, and ensuring compliance with regulations, data wrangling empowers organizations to make faster, more informed decisions. As we look to the future, the evolution of data wrangling tools—driven by automation, machine learning, and user-friendly interfaces—will further enhance the efficiency and effectiveness of data-driven decision-making, making it a cornerstone of success in 2024 and beyond.
Get your data results fast and accelerate your business performance with the insights you need today.